Generative AI refers to a type of artificial intelligence that can create new content. It learns from existing data and generates text, images, or music.
Imagine a machine that can write poems, draw pictures, or compose songs. That’s generative AI. It uses complex algorithms and vast amounts of data to produce new content. This technology mimics human creativity by learning patterns and structures in data.
For example, it can read thousands of books and then write a story in the same style. Generative AI is transforming fields like art, entertainment, and marketing. It’s making it easier to create content quickly and efficiently. But how exactly does it work? Let’s explore the inner workings of this fascinating technology.
Introduction To Generative Ai
Generative AI is a branch of artificial intelligence that focuses on creating new content. It can produce text, images, music, and even videos. This technology mimics human creativity and generates unique outputs.
Definition And Importance
Generative AI refers to AI systems that can create new content. These systems learn patterns from existing data and use this knowledge to generate new, similar data. The most important aspect of Generative AI is its ability to create original content that is often indistinguishable from human-made content.
The importance of Generative AI spans across various fields. In the entertainment industry, it can create realistic graphics and animations. In healthcare, it can generate synthetic data for research. Businesses use it to create personalized content for marketing. This technology is transforming how we create and consume content.
Brief History
The concept of Generative AI dates back to the 1950s. Early AI systems were simple and focused on rule-based approaches. These systems could only perform tasks they were explicitly programmed to do.
In the 1980s and 1990s, machine learning algorithms improved. These algorithms allowed AI systems to learn from data. The rise of neural networks in the 2000s marked a significant advancement. Neural networks mimic the human brain’s structure and learn complex patterns.
In recent years, deep learning has taken center stage. Deep learning uses large neural networks with many layers. These networks can learn intricate patterns and generate high-quality content. Technologies like GPT-3 and GANs (Generative Adversarial Networks) are examples of advanced Generative AI systems. They have achieved remarkable results in various applications.
Year | Development |
---|---|
1950s | Early AI systems with rule-based approaches |
1980s-1990s | Machine learning algorithms improve |
2000s | Rise of neural networks |
Recent years | Advancements in deep learning and Generative AI |
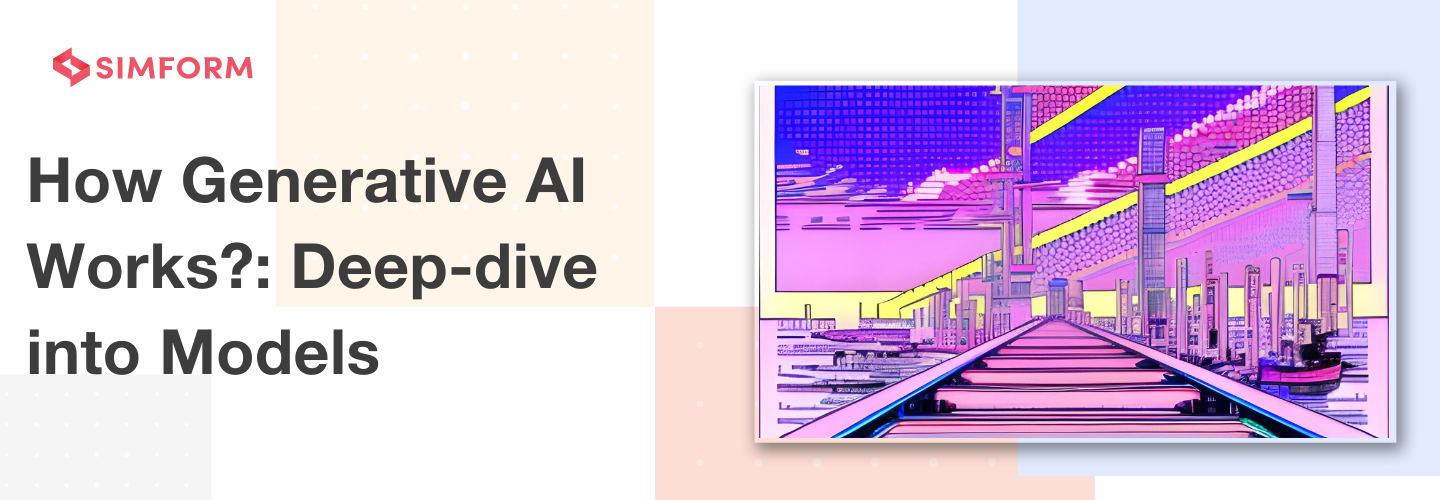
Credit: www.simform.com
Key Technologies Behind Generative Ai
Generative AI is transforming various industries by creating new and innovative solutions. The magic behind generative AI lies in its key technologies. These technologies allow machines to generate text, images, and even music. Let’s explore the main technologies behind generative AI.
Neural Networks
Neural networks are the backbone of generative AI. They mimic the human brain’s structure. Neural networks consist of layers of nodes. Each node is a simple processing unit. These nodes work together to process data. They learn from patterns in the data.
Neural networks can be trained on large datasets. This training helps the model to make accurate predictions. The more data they process, the better they become. Neural networks are used in many applications. They can recognize images, translate languages, and more.
Deep Learning
Deep learning is a subset of machine learning. It uses neural networks with many layers. These layers help in learning complex patterns. Deep learning models can handle large amounts of data. They are very effective in generating high-quality outputs.
Deep learning has revolutionized many fields. It powers voice assistants, self-driving cars, and medical diagnoses. The depth of the layers allows for greater accuracy. Deep learning models continuously improve as they process more data.
Generative AI relies heavily on deep learning. It enables machines to create realistic content. From generating human-like text to creating art, deep learning makes it possible.
Types Of Generative Models
Generative AI involves creating new data from existing data. It uses various generative models to achieve this. These models learn patterns from input data and generate similar data. Let’s explore some popular types of generative models.
Generative Adversarial Networks (gans)
Generative Adversarial Networks, or GANs, consist of two parts. The generator creates new data samples. The discriminator evaluates these samples against real data. The generator tries to fool the discriminator. The discriminator aims to distinguish between real and fake data. This process continues until the generator produces highly realistic data.
GANs are popular for creating images, videos, and music. They can generate new artwork or enhance existing images. The constant competition between the generator and discriminator improves the output quality.
Variational Autoencoders (vaes)
Variational Autoencoders, or VAEs, are another type of generative model. They consist of an encoder and a decoder. The encoder compresses input data into a smaller representation. The decoder reconstructs the data from this smaller representation. VAEs add a random element during encoding. This randomness helps generate diverse and realistic data samples.
VAEs are useful for generating images and text. They can also be used for data compression. VAEs provide a balance between data reconstruction and generation. They learn to produce new data that is similar to the input data.
How Generative Ai Works
Generative AI is a fascinating field within artificial intelligence. It creates new content, such as images, text, or music, by learning from existing data. But how does this complex technology work? Let’s break it down step-by-step.
Data Collection And Preparation
Generative AI starts with data collection. The AI needs a lot of data to learn from. This data can be images, text, or other types of content. It must be relevant to the task at hand. For example, if the AI will generate text, it needs many text samples.
Once the data is collected, it needs to be prepared. This involves cleaning the data and removing any errors or duplicates. The data is then organized in a way that the AI can understand. This step is crucial for the AI to learn effectively.
Model Training
After the data is prepared, the AI model is trained. This involves feeding the data into the AI and allowing it to learn patterns. The AI uses algorithms to understand the structure of the data. It creates a model based on these patterns.
The training process can take a long time. The AI needs to go through many iterations to learn accurately. The model is fine-tuned to improve its performance. This ensures that the AI can generate high-quality content.
Applications Of Generative Ai
Generative AI is not just a buzzword. It has practical applications in various fields. From creating stunning artworks to advancing medical research, generative AI is a powerful tool. Let’s explore some of its key applications.
Art And Design
Generative AI can create incredible art. Artists use AI to generate new ideas. It helps in creating unique designs and patterns. Fashion designers use AI to come up with fresh styles. Architects use it to visualize buildings. Graphic designers benefit from AI-generated images. The possibilities are endless.
Healthcare
Generative AI plays a vital role in healthcare. It helps in drug discovery. AI can predict the effects of new drugs. It aids in creating personalized treatment plans. AI analyzes medical images to detect diseases early. It supports doctors in making accurate diagnoses. Generative AI improves patient care and outcomes.

Credit: techjobs.sulekha.com
Benefits Of Generative Ai
Generative AI creates new content by learning from existing data. It works by using algorithms to understand patterns. This technology can generate text, images, and even music.
Generative AI offers several benefits across various fields. From improving efficiency to reducing costs, it has become a valuable tool for many businesses. This section will explore the key benefits of Generative AI.Efficiency And Creativity
Generative AI can boost efficiency in many tasks. It automates repetitive jobs, freeing up time for more important work. For instance, it can generate text, create images, and compose music. This allows humans to focus on creative tasks.Task | AI Capability |
---|---|
Text Generation | Writes articles, summaries, and reports |
Image Creation | Designs logos, graphics, and illustrations |
Music Composition | Creates melodies and harmonies |
Cost Reduction
Generative AI helps in reducing costs. It minimizes the need for manual labor. This leads to lower operational expenses. Businesses save on salaries, training, and other overheads.- Automated content creation lowers the need for writers.
- AI-designed graphics reduce the need for graphic designers.
- AI-driven customer service cuts down on support staff.
Challenges And Limitations
Generative AI is a fascinating field, but it comes with challenges and limitations. These issues can impact the effectiveness and ethical use of AI. Understanding these challenges is crucial for responsible AI development and deployment.
Ethical Concerns
One major challenge is the ethical use of generative AI. AI can create realistic images, texts, and videos. This can lead to misuse, such as spreading misinformation. AI-generated content can also raise privacy issues. Sensitive data can be replicated without consent.
Bias is another ethical concern. AI models learn from existing data. If the data contains biases, the AI will replicate them. This can lead to unfair or discriminatory outcomes. Ensuring fairness and transparency in AI is vital.
Technical Barriers
Generative AI also faces technical barriers. One issue is the high computational cost. Training AI models requires significant resources. This can be expensive and time-consuming.
Another technical challenge is maintaining quality. AI-generated content can sometimes lack consistency. Ensuring high-quality outputs is a constant hurdle. Fine-tuning models to improve performance is necessary.
Scalability is a concern too. As the demand for AI grows, systems need to handle larger datasets. This requires robust infrastructure and expertise. Overcoming these technical barriers is essential for broader AI adoption.
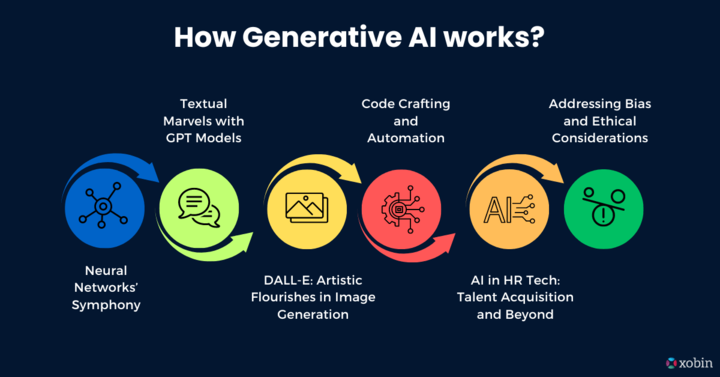
Credit: xobin.com
Future Of Generative Ai
The future of generative AI looks promising and full of potential. As technology advances, generative AI continues to evolve, offering new possibilities. This section explores the emerging trends and long-term impact of generative AI.
Emerging Trends
Generative AI is becoming more sophisticated and accessible. New algorithms and models are constantly being developed. These improvements lead to better performance and more realistic outputs. One trend is the use of generative AI in creative fields. Artists and designers use AI to generate unique artwork and designs. Another trend is AI-generated content in media. AI can write articles, create music, and even produce videos.
Businesses are also adopting generative AI for various applications. From marketing to product development, AI helps streamline processes. AI-generated content can personalize customer experiences, making them more engaging. Healthcare is another field where generative AI shows promise. AI can generate synthetic medical data for research and training. This helps improve healthcare outcomes and patient care.
Long-term Impact
The long-term impact of generative AI is significant. It has the potential to transform many industries. One impact is the democratization of creativity. AI tools make it easier for anyone to create art, music, and content. This can lead to a surge in creative output and diversity.
Generative AI also poses challenges. Ethical concerns arise around AI-generated content. Issues like authorship, originality, and bias need addressing. Ensuring AI-generated content is fair and unbiased is crucial. Another impact is the potential for job displacement. As AI takes over certain tasks, some jobs may become obsolete. However, new opportunities will also emerge. The key is to adapt and prepare for these changes.
Education and training will play a vital role in this transition. People need to learn new skills to work alongside AI. Emphasizing creativity and critical thinking will be essential. The future of generative AI holds great promise and potential. The journey is just beginning, and the possibilities are endless.
Frequently Asked Questions
What Is Generative Ai?
Generative AI is a type of artificial intelligence that creates content. It can generate text, images, music, and more. It uses algorithms and deep learning models to produce new data.
How Does Generative Ai Work?
Generative AI works by learning patterns in data. It uses neural networks and algorithms to generate new content. It creates outputs that are similar to its training data.
What Are Examples Of Generative Ai?
Examples of Generative AI include GPT-3, DALL-E, and DeepArt. These models generate text, images, and art. They showcase the creative capabilities of AI.
Why Is Generative Ai Important?
Generative AI is important because it enhances creativity and efficiency. It automates content creation and design tasks. It also drives innovation in various fields.
Conclusion
Generative AI creates new content by learning from existing data. It finds patterns and generates text, images, or sounds. This technology powers chatbots, art creation, and music composition. Understanding its basics helps us appreciate its potential. Remember, AI tools are here to assist, not replace.
As AI evolves, more applications will emerge. Stay curious and keep learning about this fascinating field.