Natural Language Processing (NLP) is a key part of AI. It helps computers understand human language.
But how does NLP actually work in AI? NLP involves teaching machines to read, interpret, and respond to human language. This process allows AI systems to perform tasks like translation, sentiment analysis, and even conversation. By breaking down language into manageable parts, NLP enables AI to recognize patterns and meanings.
This makes it possible for machines to interact with us in a more natural way. Understanding NLP’s role in AI helps us appreciate the technology behind chatbots, virtual assistants, and more. Dive in to explore the fascinating workings of NLP in AI.
Core Components
Understanding the core components of Natural Language Processing (NLP) is essential. These components help machines understand human language. Let’s dive into two critical parts: Tokenization and Syntax Analysis.
Tokenization
Tokenization is the first step in NLP. It breaks down text into smaller pieces called tokens. These tokens can be words or phrases. Tokenization helps in understanding the text better.
There are different types of tokenization:
- Word Tokenization: Splits text into individual words.
- Sentence Tokenization: Divides text into sentences.
- Character Tokenization: Breaks text into characters.
For example, the sentence “NLP is fun!” can be tokenized into:
Type | Tokens |
---|---|
Word Tokenization | NLP, is, fun |
Sentence Tokenization | NLP is fun! |
Character Tokenization | N, L, P, , i, s, , f, u, n |
Syntax Analysis
Syntax Analysis is another vital component of NLP. It involves analyzing the grammatical structure of sentences. Syntax Analysis helps in understanding relationships between words.
Key tasks in Syntax Analysis include:
- Part-of-Speech Tagging: Identifying the parts of speech (nouns, verbs, etc.) in a sentence.
- Parsing: Creating a tree structure that represents the sentence’s grammatical structure.
Consider the sentence “The cat sat on the mat.” Syntax Analysis breaks it down like this:
- Noun Phrase: The cat
- Verb Phrase: sat on the mat
This analysis helps in understanding how words are related and structured.
Key Techniques
Natural Language Processing (NLP) is a crucial part of AI. It allows machines to understand and interpret human language. There are several key techniques in NLP that make this possible. Two of the most important are Named Entity Recognition and Sentiment Analysis. Let’s explore them in detail.
Named Entity Recognition
Named Entity Recognition (NER) helps identify and classify key information in text. It finds names of people, organizations, places, and more. For example, in the sentence “Apple was founded by Steve Jobs in California,” NER identifies “Apple” as a company, “Steve Jobs” as a person, and “California” as a location. This technique helps in organizing and structuring unstructured data.
NER uses algorithms and models to detect entities. It often relies on machine learning and statistical methods. Training the model with a large dataset improves its accuracy. NER is useful in many applications, from search engines to chatbots.
Sentiment Analysis
Sentiment Analysis determines the emotional tone behind a text. It helps understand if the sentiment is positive, negative, or neutral. For instance, “I love this product” has a positive sentiment, while “I hate waiting” has a negative sentiment. This technique is widely used in customer feedback and social media monitoring.
Sentiment Analysis uses various methods. It can rely on machine learning, where models learn from labeled data. Or it can use lexicon-based approaches, which involve predefined lists of words. The goal is to understand the user’s feelings and opinions.
Both Named Entity Recognition and Sentiment Analysis play vital roles in NLP. They help machines understand and process human language better. These techniques are integral in making AI applications more effective and user-friendly.
Machine Learning In Nlp
Machine Learning plays a crucial role in Natural Language Processing (NLP). It helps machines understand and process human language. Two main types of machine learning are used in NLP: Supervised Learning and Unsupervised Learning. Each type has its unique approach and benefits.
Supervised Learning
In Supervised Learning, the machine gets trained using labeled data. This data includes input-output pairs. For example, a sentence with its correct translation. The machine learns from this data. It creates a model that predicts the output for new inputs. This method is useful for tasks like sentiment analysis. It can determine if a text is positive or negative.
Unsupervised Learning
Unsupervised Learning does not use labeled data. Instead, it finds patterns in the data on its own. The machine looks for similarities and groups the data accordingly. This method is used for tasks like topic modeling. It helps identify the main topics in a set of documents. Unsupervised Learning is useful for discovering hidden structures in data.
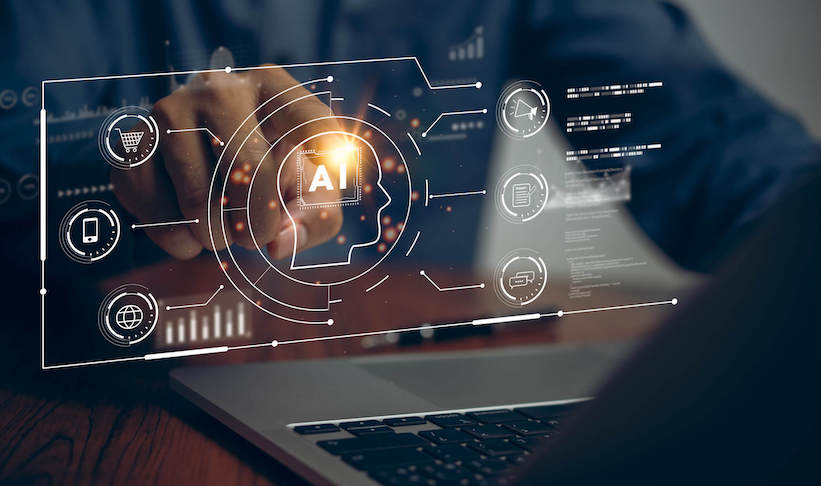
Credit: onlinedegrees.sandiego.edu
Deep Learning Approaches
Deep learning is a subset of artificial intelligence. It uses complex algorithms to mimic the human brain. In natural language processing (NLP), deep learning plays a crucial role. It helps machines understand and generate human language. Let’s explore two key deep learning approaches in NLP: Neural Networks and Transformers.
Neural Networks
Neural networks are the backbone of deep learning. They consist of layers of nodes, or “neurons.” Each neuron processes a part of the input data. This structure allows neural networks to recognize patterns in data.
In NLP, neural networks help in tasks like text classification and sentiment analysis. They can learn from large amounts of text data. Here is a simple structure of a neural network:
Layer | Function |
---|---|
Input Layer | Receives the raw data |
Hidden Layers | Processes the data |
Output Layer | Generates the result |
Neural networks are powerful in handling complex language tasks. They are versatile and can be used in various NLP applications.
Transformers
Transformers are a recent advancement in deep learning. They have revolutionized NLP. Transformers use a mechanism called attention. This allows them to focus on different parts of the input data.
Transformers excel in tasks like language translation and text generation. They can handle long sentences better than traditional models. Here are some key features of transformers:
- Self-attention mechanism
- Parallel processing
- Scalability
One popular transformer model is BERT (Bidirectional Encoder Representations from Transformers). BERT has set new benchmarks in NLP tasks. It understands the context of words in sentences better.
In summary, both neural networks and transformers are essential in NLP. They help machines understand and generate human language effectively.
Applications Of Nlp
Natural Language Processing (NLP) is a crucial part of Artificial Intelligence (AI). It helps machines understand and interact with human language. NLP has a wide range of applications in various fields. Below, we explore some key applications of NLP that are transforming the way we interact with technology.
Chatbots
Chatbots use NLP to understand and respond to user queries. They can hold conversations, answer questions, and provide support. Companies use chatbots for customer service and online assistance. They are available 24/7 and can handle multiple inquiries at once. This improves efficiency and customer satisfaction.
Language Translation
Language translation tools rely on NLP to convert text from one language to another. These tools make communication easier across different languages. They help in translating documents, websites, and even real-time conversations. This is crucial for businesses operating in global markets. It also helps individuals communicate while traveling.
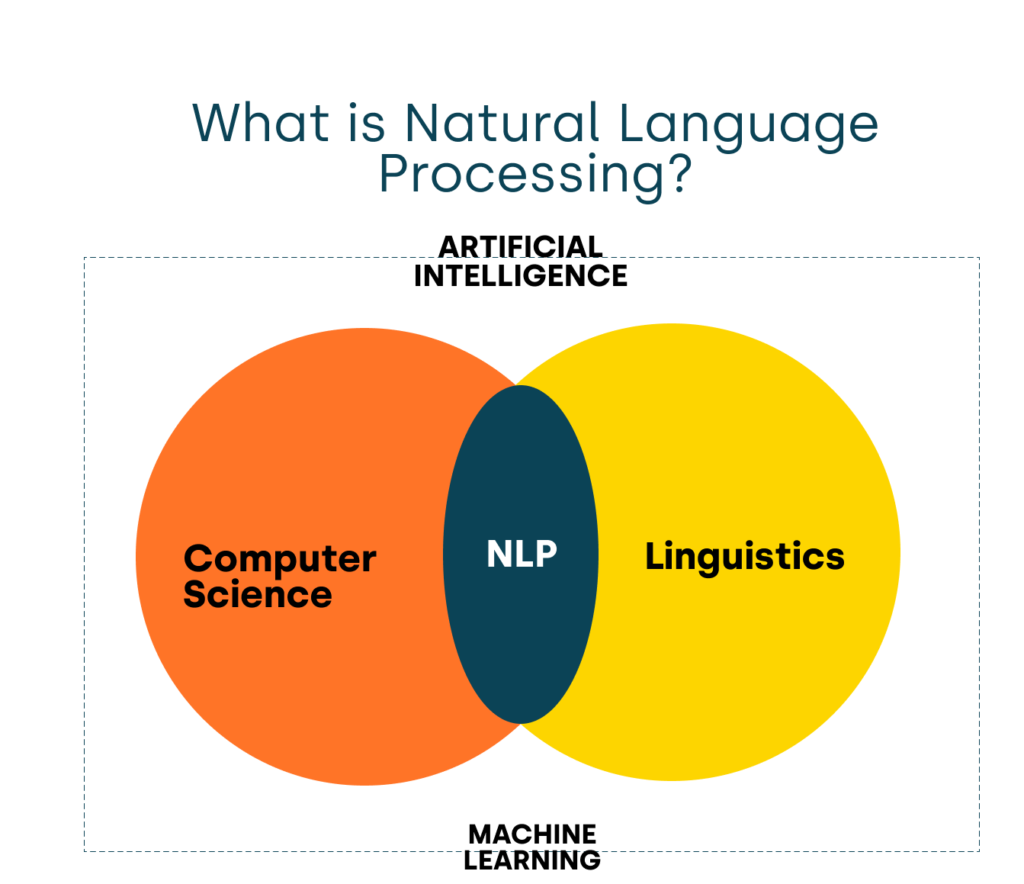
Credit: www.qtravel.ai
Challenges In Nlp
Natural Language Processing (NLP) is essential in AI. NLP helps machines understand human language. Despite its progress, NLP faces many challenges. These challenges hinder perfect language understanding.
Ambiguity
Ambiguity in language is a major issue. Words often have multiple meanings. For instance, “bank” can mean a financial institution or the side of a river. This makes it hard for AI to choose the right meaning. Sentences, too, can be ambiguous. “He saw the man with a telescope” can be interpreted in different ways. It is difficult for AI to resolve such ambiguities without context.
Context Understanding
Context plays a crucial role in language. Words and sentences have different meanings in different contexts. AI often struggles to grasp these contextual nuances. For example, “bat” can refer to an animal or sports equipment. The intended meaning depends on the surrounding text. Understanding sarcasm and idioms also requires context. AI needs advanced algorithms to decode these subtleties.
Future Of Nlp
Natural Language Processing (NLP) is a fast-evolving field in AI. Its future promises exciting advancements and applications. Understanding these changes helps us prepare for what’s next in technology.
Advancements
Recent years have seen remarkable advancements in NLP. With better algorithms and more data, models are now more accurate. Here are some key advancements:
- Transformers: These models can handle more complex tasks.
- BERT and GPT: These are powerful pre-trained models that have set new benchmarks.
- Multilingual NLP: These systems can understand and translate multiple languages.
These improvements help NLP applications perform better in various areas. For example, chatbots, translation services, and sentiment analysis are becoming more reliable.
Ethical Considerations
As NLP technology evolves, ethical concerns arise. Ensuring fairness and avoiding bias are critical. Here are some ethical considerations:
Issue | Explanation |
---|---|
Bias | Models may reflect societal biases present in the data. |
Privacy | Collecting and using data responsibly is essential. |
Transparency | Users should understand how decisions are made by NLP systems. |
Addressing these issues ensures that NLP technology benefits all users fairly and responsibly.
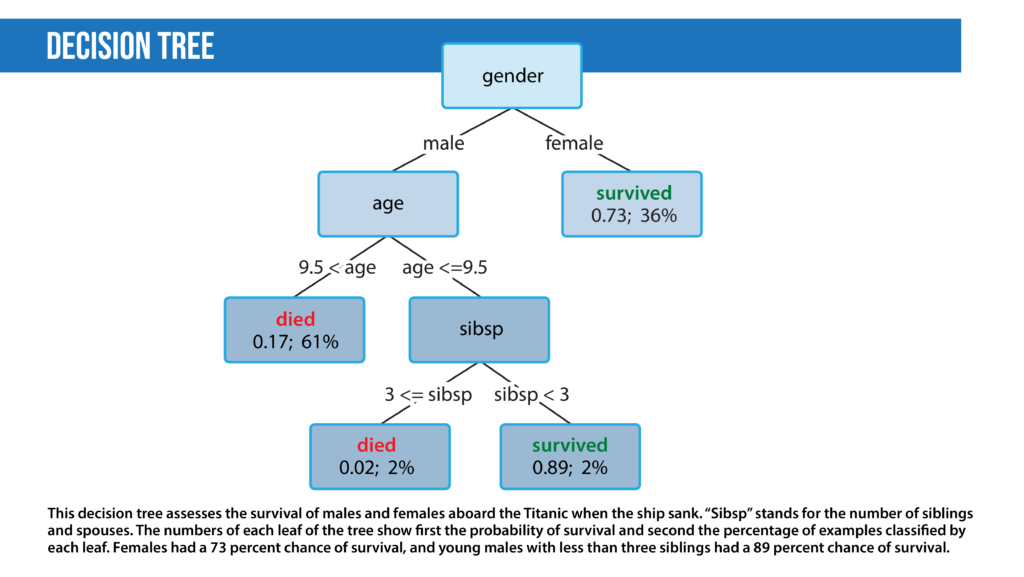
Credit: www.deeplearning.ai
Frequently Asked Questions
What Is Natural Language Processing In Ai?
Natural Language Processing (NLP) is a field of AI that focuses on the interaction between computers and humans using natural language. It helps machines understand, interpret, and respond to human language.
How Does Nlp Work In Ai?
NLP in AI works by using algorithms to analyze and understand human language. It involves processes like tokenization, parsing, and sentiment analysis to derive meaning from text.
What Are Common Applications Of Nlp?
Common applications of NLP include chatbots, language translation, sentiment analysis, and voice assistants. These applications improve human-computer interaction by understanding and processing human language.
Why Is Nlp Important In Ai?
NLP is important in AI because it enables machines to understand and respond to human language. This enhances user experience and makes technology more accessible.
Conclusion
Natural Language Processing (NLP) is a key part of AI technology. It helps machines understand human language. NLP can analyze text, translate languages, and even chat with users. Businesses use NLP to improve customer service and automate tasks. As AI grows, NLP will become more important.
Understanding NLP helps us see how AI can assist in daily life. This technology bridges the gap between human communication and machines. Stay curious and explore how NLP can benefit you.