AI learns from data by identifying patterns and making predictions. It uses algorithms to process large datasets and improve over time.
Understanding how AI learns from data is key to grasping its capabilities. AI, or Artificial Intelligence, relies on data to make decisions and solve problems. The process involves feeding data into AI systems, which then use algorithms to find patterns.
These patterns help AI to predict outcomes and make informed choices. As more data is processed, AI systems become smarter and more accurate. This continuous learning loop is what makes AI so powerful. By grasping this concept, you can better appreciate the potential and limitations of AI in various fields. So, let’s delve deeper into how AI learns from data and why it matters.
Introduction To Ai Learning
Artificial Intelligence (AI) is transforming our world. It’s making machines smarter and more capable. But how does AI learn from data? Understanding this can help demystify AI. In this section, we will explore the basics of AI learning. We will also discuss the role of data in this process.
What Is Ai?
AI, or Artificial Intelligence, is the simulation of human intelligence by machines. It enables machines to perform tasks that usually require human intellect. These tasks include problem-solving, learning, and decision-making. AI can be found in various applications, from voice assistants to self-driving cars.
Importance Of Data In Ai
Data plays a crucial role in AI learning. AI systems rely on vast amounts of data to learn and improve. Data acts as the fuel that powers AI algorithms. The more data an AI system has, the better it can learn and make accurate predictions.
In AI learning, data is used to train algorithms. These algorithms analyze the data and identify patterns. This helps the AI system to make decisions and predictions. Without data, AI would not be able to function effectively.
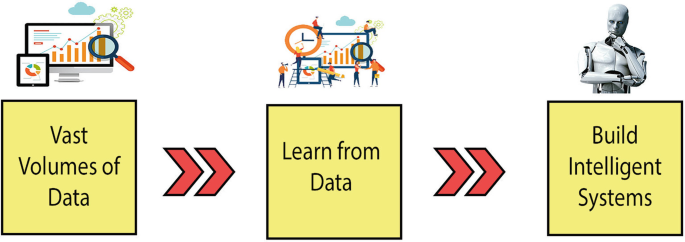
Credit: link.springer.com
Types Of Learning In Ai
Artificial Intelligence (AI) learns from data through different methods. These methods are called types of learning in AI. Each type helps AI understand and process data in unique ways. Let’s explore these types of learning: supervised learning, unsupervised learning, and reinforcement learning.
Supervised Learning
Supervised learning involves a teacher guiding the AI. The teacher provides labeled data. Each data point has a correct answer. The AI learns to predict the answer for new data. For example, a dataset of cat and dog images with labels helps AI distinguish between cats and dogs. The AI learns from the examples to make accurate predictions.
Unsupervised Learning
Unsupervised learning has no teacher. The AI gets unlabeled data. The AI must find patterns on its own. It groups similar data points together. This type of learning is useful for clustering and association tasks. For example, an AI can group customer data to identify different market segments.
Reinforcement Learning
Reinforcement learning is like training a pet. The AI learns through trial and error. It gets rewards for good actions and penalties for bad actions. The goal is to maximize rewards. This type of learning is common in game playing. For example, AI can learn to play chess by trying different moves and learning from the outcomes.
Data Collection Methods
Data collection is the first step in teaching AI. It’s crucial for AI’s learning process. Without data, AI cannot learn or make decisions. There are different methods to gather data. Each method has its own benefits and drawbacks. In this section, we will explore some common data collection methods. We will also discuss the importance of data quality and quantity.
Sources Of Data
AI needs data from various sources to learn effectively. One common source is user-generated content. This includes social media posts, reviews, and comments. Another source is sensor data. Sensors in devices like smartphones and cars collect valuable information. Websites and online platforms also provide data. They track user behavior and interactions. Public datasets are another option. These are available from research institutions and governments.
Data Quality And Quantity
Quality and quantity of data are key to AI’s success. High-quality data is accurate and relevant. It should be free from errors and biases. Poor quality data leads to incorrect predictions. On the other hand, quantity of data is equally important. More data helps AI learn better. It improves the model’s accuracy and performance. But it’s not just about having more data. The data must be diverse and comprehensive. This ensures the AI can handle different scenarios.
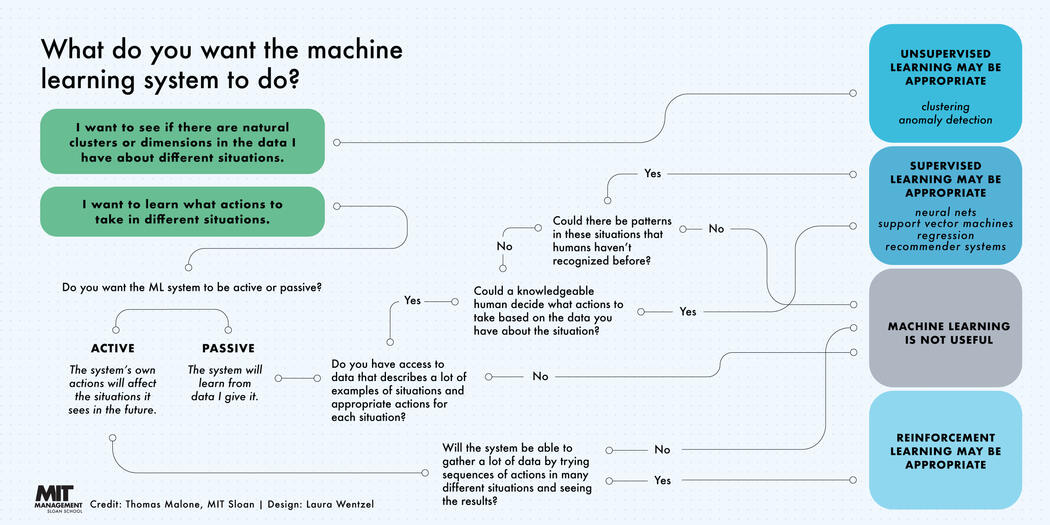
Credit: mitsloan.mit.edu
Data Preprocessing Techniques
Before AI can learn from data, it needs to be prepared. This preparation is called data preprocessing. It is a crucial step to ensure the AI model learns accurately. Let’s dive into some key data preprocessing techniques.
Cleaning Data
Data often comes with noise, missing values, or errors. Cleaning data is about removing these issues. Here are some common steps:
- Remove duplicates: Duplicate entries can skew results.
- Handle missing values: Fill them with averages or discard them.
- Correct errors: Fix typos and incorrect data points.
Clean data is essential for accurate AI learning.
Normalizing Data
Data points can have different scales. Normalizing data brings all values into a similar range. This helps the AI model understand the data better.
- Min-Max Scaling: Converts data to a range between 0 and 1.
- Z-score Normalization: Converts data to have a mean of 0 and a standard deviation of 1.
Normalizing data ensures consistent learning by the AI model.
Feature Engineering
Feature engineering involves creating new features or modifying existing ones. These features help the AI model understand the data better.
- Create new features: Combine or transform existing data points.
- Select important features: Choose features that have a significant impact.
- Reduce dimensionality: Use techniques like PCA to reduce the number of features.
Effective feature engineering can greatly improve the AI model’s performance.
Training Ai Models
Training AI models is a crucial step in the AI development process. It involves teaching the AI system to perform tasks by learning from data. This process helps the AI understand patterns, make predictions, and improve its accuracy over time. Let’s explore the key aspects of training AI models.
Model Selection
Model selection is the first step in training AI. It involves choosing the right model for the specific task. Different models suit different types of data and problems. For example, neural networks are great for image recognition. Decision trees work well for classification tasks. Selecting the right model is essential for achieving good results.
Training Algorithms
Training algorithms play a vital role in teaching AI models. They adjust the model’s parameters to fit the data. Popular algorithms include gradient descent and backpropagation. These algorithms help the model learn from errors. They minimize the difference between the predicted and actual outcomes. This process is iterative and requires multiple passes through the data.
Evaluation Metrics
Evaluation metrics measure the performance of AI models. They help determine how well the model is learning. Common metrics include accuracy, precision, and recall. Accuracy measures the percentage of correct predictions. Precision indicates the proportion of true positive results. Recall shows the ability to identify all relevant instances. These metrics provide insights into the model’s strengths and weaknesses.
Challenges In Ai Learning
AI learning is not a smooth ride. There are many bumps along the way. Challenges in AI learning can impact its performance and reliability. These challenges need careful handling to build effective AI models.
Overfitting And Underfitting
Overfitting happens when an AI model learns data details too well. It performs well on training data but fails on new data. This means the model is too specific. On the other hand, underfitting occurs when the model is too simple. It cannot capture the data patterns. This leads to poor performance on both training and new data.
Bias And Variance
Bias and variance are two sides of the same coin. High bias means the model has a strong assumption. It may miss relevant data trends. High variance means the model is very sensitive to data. It performs well on training data but poorly on new data. Balancing bias and variance is crucial. This creates a model that generalizes well.
Data Privacy Concerns
Data privacy is a big concern in AI learning. AI models need large amounts of data. This data often includes personal information. Protecting this information is vital. Breaches can lead to trust issues and legal problems. Ensuring data privacy requires careful handling and compliance with regulations.
Applications Of Ai Learning
AI learning has a wide range of applications. From improving healthcare to optimizing finance, AI is transforming many industries. Each application shows the power and potential of AI learning. Let’s explore some key areas where AI makes a big impact.
Healthcare
AI helps doctors diagnose diseases faster. It analyzes medical images and finds patterns. This leads to early detection of issues like tumors. AI also predicts patient outcomes. It uses data from past treatments to suggest best practices. AI assists in drug discovery. It speeds up the process by simulating trials and analyzing results. AI in healthcare means better care and faster recovery.
Finance
AI protects against fraud. It monitors transactions for unusual activity. This helps banks catch fraud before it happens. AI also improves customer service. Chatbots answer questions and solve problems quickly. AI helps in investment decisions too. It analyzes market trends and predicts stock movements. AI in finance means safer transactions and smarter investments.
Transportation
AI makes driving safer. Self-driving cars use AI to navigate roads. They avoid accidents by detecting obstacles. AI also improves traffic flow. It analyzes data from sensors to manage traffic lights. This reduces congestion. AI in transportation means safer roads and smoother commutes.
Future Of Ai Learning
The future of AI learning is both exciting and complex. AI continues to evolve, adapt, and expand its capabilities. Understanding the trends, ethical concerns, and potential impacts is crucial for anyone interested in this field.
Emerging Trends
AI learning is moving towards more advanced and autonomous systems. The following trends are shaping the future of AI learning:
- Deep learning: AI systems using deep neural networks to learn complex patterns.
- Reinforcement learning: AI learns by interacting with its environment and receiving feedback.
- Transfer learning: AI uses knowledge from one task to improve learning in another task.
Ethical Considerations
As AI grows, ethical considerations become more critical. Key issues include:
- Bias: AI can inherit biases from the data it learns from. Ensuring fairness is essential.
- Privacy: AI systems often require vast amounts of data, raising privacy concerns.
- Accountability: Determining who is responsible for AI decisions is important.
Potential Impacts
The impact of AI learning on society can be significant. Some potential impacts include:
- Job displacement: Automation may replace certain jobs, requiring new skills.
- Healthcare improvements: AI can enhance diagnostics and treatment plans.
- Environmental monitoring: AI can help track and predict environmental changes.
Understanding these aspects will help us navigate the future of AI learning effectively.
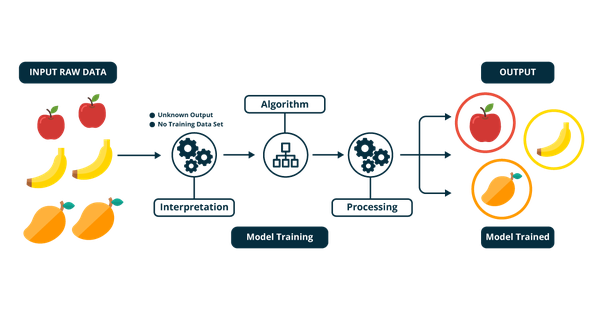
Credit: www.onemodel.co
Frequently Asked Questions
What Is Ai Learning From Data?
AI learns from data by identifying patterns and making predictions. It uses algorithms to process and analyze the data to improve its performance over time.
How Does Machine Learning Use Data?
Machine learning uses data to train algorithms. The data helps the model learn and make accurate predictions or decisions based on new inputs.
What Types Of Data Does Ai Use?
AI uses structured and unstructured data. Structured data is organized and easy to analyze, while unstructured data includes text, images, and videos.
Why Is Data Important For Ai?
Data is crucial for AI because it provides the information needed to learn and improve. Without data, AI models cannot be trained effectively.
Conclusion
AI learns from data through patterns and algorithms. It improves with more data. This process makes AI smarter. Understanding this helps in everyday tech use. The future of AI is promising. With data, AI enhances our lives. Thanks for exploring this topic with us.
Stay curious and keep learning.